Learner models hold and dynamically update the information
about a user’s learning: current knowledge, competencies,
misconceptions, goals, affective states, etc. There is an
increasing trend towards opening the learner model to the
user (learner, teacher or other stakeholders), often to support
reflection and encourage greater learner responsibility for
their learning; as well as helping teachers to better understand
their students (Bull & Kay, 2010). A core requirement
is that such visualisations must be understandable to the
user. On the surface this may appear similar to the more recent
work on learning analytics. However, open learner models (OLM)
focus much more on the current state of learners, and with
less reference to activities undertaken, scores gained, materials
used, contributions made, etc. OLMs typically focus more on
concepts or competencies, to guide learners towards consideration
of conceptual issues rather than specific activities and performance.
Various OLM visualisation examples have been described in
the literature for university students (see Bull & Kay
2010, for a more detailed overview). The most common visualisations
used in courses include skill meters, concept maps and hierarchical
tree structures. Recently, tree map overview-zoom-filter approaches
to open learner modelling have also started to appear, as
have tag/word clouds (Bull et al., in press; Mathews et al.,
2012), and sunburst views (Conejo et al., 2011).
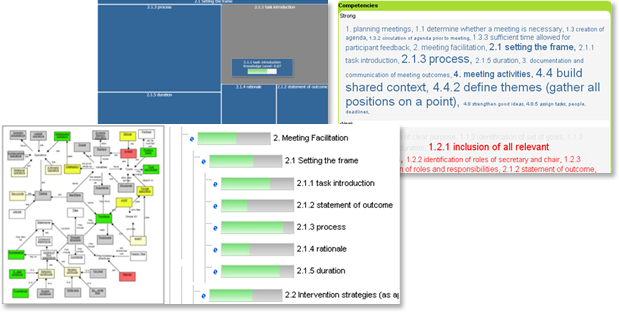 |
Girard and
Johnson (2008) aimed to extend considerations about
the presentation of OLMs to school level. To date, at
that level most attempts have been very simple in presentation,
a common example being smileys or proficiency indicated
by colour (e.g. in Khan Academy, 2012). Other recent
approaches include the many new learning analytics dashboards
(e.g. Verbert et al., 2013), though these are not typically
based on learner modelling. Research has considered
whether some learner model visualisations may be more
suitable than others. For example: a recent eye-tracking
study of university students trying to understand four
learner model presentations - kiviat chart, concept
tag cloud, tree map and concept hierarchy - found the
kiviat graph and concept hierarchy to be more efficient
(for understanding the representation), than the tree
map and tag cloud (Mathews et al., 2012).
|
Furthermore, the kiviat chart was considered to be the best
format through which to gain a quick overview of knowledge;
although other views were useful if further detail was required.
Based on these results, Mathews et al (2012) conclude that
the most useful visualisation is likely to depend on the context
for which it is being used. Another OLM eye-tracking study
found that, when concept map, pre-requisite map, tree structure
of concepts, tree structure following lecture topics and sub-topics,
and alphabetical index of the same underlying model were compared,
visual attention in a view depended on whether the view was
amongst the user’s preferred views, rather than on the
structure of the view itself (Bull et al., 2007). Albert et
al. (2010) state that university students thought simpler
views were understandable and suitable for gaining an overview
of their learning; while a more complex activity visualisation
was less popular, perhaps because the complexity of the information
was difficult to understand. Duan et al (2010) found preferences
towards skill meters over more complex visualisations; however,
users with more complex views of the learner model perceived
it to be more accurate. Also, although a majority, the preference
for the simpler skill meters was still only 53%. Ahmad and
Bull (2008) also found that students perceived more detailed
views of the learner model to be more accurate than skill
meters. However, users still reported a higher level of trust
in the more simple skill meter overviews. University students
with experience with a range of different OLM systems have
indicated their preference for having both overviews and detailed
learner model information available for viewing (Bull, 2012).
In addition to
visualising the learner model, various methods of interacting
with the learner model exist, ranging from simple inspectable
models, through those that allow some kind of additional
evidence to be input directly by users, to negotiated
learner models, where the content of the learner model
is discussed and, as a result, potentially updated. The
latter is our focus here. Key features of negotiated learner
models are, therefore, not only that the presentation
of the learner model must be understandable by the user,
but also that the aim of the interactive learner modelling
should be an agreed model. Most negotiated learner models
are negotiated between the student and a teaching system.
However, other stakeholders can also be involved, and
the notion of “the system” can be broadened
to include a range of technologies such as used in technology-enhanced
learning. We here consider (i) fully negotiated learner
models; (ii) partially negotiated learner models; and
(iii) other types of learner model discussion. These are
all relevant to our notion of negotiating about the learner
model, or negotiating its content, as proposed for LEA’s
BOX. |
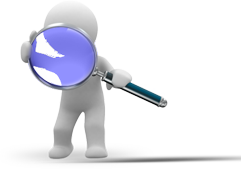
|
Mr Collins was the first learner model designed for negotiation
with the student (Bull & Pain, 1995). Its focus was on
increasing model accuracy by student-system discussion of
the model, while at the same time promoting learner reflection
through discussion. The model contained separate belief measures:
the system’s inferences about the student’s understanding,
and the student’s own confidence in their knowledge,
with identical interaction moves available to learner and
system to try to resolve any discrepancies (e.g. challenge,
offer evidence). Subsequent work extended the types of negotiation
available, from the original menu-based discussion, to include
dialogue games (Dimitrova, 2003) and chatbot (Kerly &
Bull, 2008).
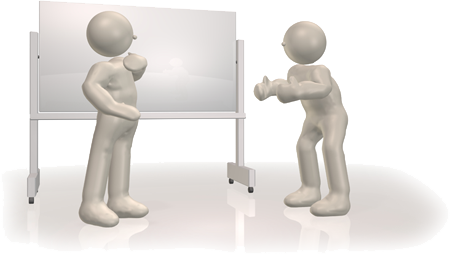 |
Close to the above definition of negotiated
learner models is xOLM (Van Labeke et al., 2007). However,
xOLM relied on the student to initiate discussion of
the model. For example, students can challenge claims,
warrants and backings, and receive justifications from
the system. Learners can choose to agree, disagree,
or move on (without resolution). Unlike full negotiation,
the system allowed the learner's challenge to succeed
where there is unresolved disagreement. In contrast,
EI-OSM defers the overriding decision to the (human)
teacher if interaction between a student and teacher
cannot resolve discrepancies using the system’s
evidence-based argument approach (Zapata-Rivera et al.,
2007). There were mixed reactions from teachers as to
whether they would consider assessment claims from students
without the availability of relevant evidence, but they
believed that these could form a useful starting point
for formative dialogue.
|
While not a negotiated learner model, a Facebook group to
allow university students to discuss their learner models
with each other (Alotaibi & Bull, 2012), indicating a
willingness to critically consider understanding in an open-ended
way. This is crucial for methods of model negotiation between
human partners where open discussion is encouraged. A similar
approach in the sense that the model itself is not negotiated,
is allowing children to provide their assessments of their
knowledge to the system if they disagree with it, quantitatively
and explained in text comments which can be seen by the teacher.
Such input can become a focus for subsequent (human) teacher-child
discussion (Zapata-Rivera & Greer, 2004). The NEXT-TELL
CoNeTo tool (Vatrapu et al., 2012) offers a socio-cultural
approach to negotiating the learner model, which allows learners
and teachers to discuss the NEXT-TELL OLM (Bull et al., in
press), while focussing on artefacts and evidence. In LEA’s
BOX we aim to incorporate evidence-based discussion and learner
model negotiation, at the level appropriate to age, STEM subject
(with reference to competencies), and learning goals, as identified
by teachers.
|
|
References
Ahmad, N. & Bull, S. (2008). Do Students Trust their Open Learner
Models?, In W. Neijdl, J. Kay, P. Pu and E. Herder (Eds). Adaptive
Hypermedia and Adaptive Web-Based Systems (pp. 255-258), Berlin
Heidelberg: Springer-Verlag.
Albert, D., Nussbaumer, A. & Steiner, C.M. (2010). Towards Generic
Visualisation Tools and Techniques for Adaptive E-Learning, In S.L.
Wong et al. (Eds). International Conference on Computers in Education
(pp. 61-65), Putrajaya, Malaysia, Asia-Pacific Society for Computers
in Education.
Bull, S. (2012). Preferred Features of Open Learner Models for University
Students, In S.A. Cerri, W.J. Clancey, G. Papadourakis and K. Panourgia
(Eds.), Intelligent Tutoring Systems (pp. 411-421), Berlin- Heidelberg:
Springer-Verlag.
Bull, S., Cooke, N. & Mabbott, A. (2007). Visual Attention in
Open Learner Model Presentations: An Eye-Tracking Investigation,
In C. Conati, K. McCoy and G. Paliouras (Eds.), User Modeling (pp.
177-186), Berlin-Heidelberg: Springer-Verlag.
Bull, S., Johnson, M., Alotaibi, M., Byrne, W. & Cierniak, G.
(in press). Visualising Multiple Data Sources in an Independent
Open Learner Model, In H.C. Lane and K. Yacef (Eds.), Artificial
Intelligence in Education, Berlin-Heidelberg: Springer-Verlag.
Bull, S. & Kay, J. (2010). Open Learner Models, In R. Nkambou,
J. Bordeau and R. Miziguchi (Eds.), Advances in Intelligent Tutoring
Systems (pp. 318-338), Berlin-Heidelberg: Springer-Verlag.
Bull, S. & Pain, H. (1995). 'Did I Say What I Think I Said,
And Do You Agree With Me?': Inspecting and Questioning the Student
Model, In J. Greer (Ed), Proccedings of the AIED95, (pp.501-508),
AACE, Charlottesville VA.
Conejo, R., Trella, M., Cruces, I. & Garcia, R. (2011). INGRID:
A Web Service Tool for Hierarchical Open Learner Model Visualisation,
UMAP 2011 Adjunct Poster Proceedings: Available at: http://www.umap2011.org/proceedings/posters/paper_241.pdf
Dimitrova, V. (2003). STyLE-OLM: Interactive Open learner Modelling,
International Journal of Artificial Intelligence in Education, 13(1),
35-78.
Duan, D., Mitrovic, A. & Churcher, N. (2010). Evaluating the
Effectiveness of Multiple Open Student Models in EER-Tutor, In S.L.
Wong et al. (Eds), International Conference on Computers in Education
(pp. 86-88), Putrajaya, Malaysia, Asia-Pacific Society for Computers
in Education.
Girard, S. & Johnson, H. (2008). Designing and Evaluating Affective
Open-Learner Modeling Tutors, Proceedings of Interaction Design
and Children (Doctoral Consortium, (pp. 13-16), Chicago, IL.
Khan Academy (2012). Exercise Dashboard (Knowledge Map). Available
at: http://www.khanacademy.org/exercisedashboard. (Accessed 3rd
July 2012).
Kerly, A. & Bull, S. (2008). Children's Interactions with Inspectable
and Negotiated Learner Models, In B.P. Woolf, E. Aimeur, R. Nkambou
and S. Lajoie (Eds), Intelligent Tutoring Systems: 9th International
Conference (pp. 132-141), Berlin Heidelberg: Springer-Verlag.
Mathews, M., Mitrovic, A., Lin, B., Holland, J. & Churcher,
N. (2012). Do Your Eyes Give it Away? Using Eye-Tracking Data to
Understand Students’ Attitudes Towards Open Student Model
Representations, In S.A. Cerri, W.J. Clancey, G. Papadourakis and
K. Panourgia (Eds), Intelligent Tutoring Systems (pp. 422-427),
Berlin-Heidelberg: Springer-Verlag.
Van Labeke, N., Brna, P. & Morales, R. (2007). Opening up the
Interpretation Process in an Open Learner Model, International Journal
of Artificial Intelligence in Education, 17(3), 305-338.
Vatrapu, R., Tanveer, U. & Hussain, A. (2012). Towards teaching
analytics: communication and negotiation tool (CoNeTo), Proceedings
of the 7th Nordic Conference on Human-Computer Interaction: Making
Sense Through Design, 775-776.
Verbert, K., Duval, E., Klerkx, J., Govaerts, S., Santos, J.L. (2013).
Learning Analytics Dashboard Applications, American Behavioral Scientist:
Zapata-Rivera, J-D. & Greer, J.E. (2004). Interacting with Inspectable
Bayesian Student Models, International Journal of Artificial Intelligence
in Education, 17(3), 127-163.
Zapata-Rivera, D., Hansen, E., Shute, V.J., Underwood, J.S. &
Bauer, M. (2007). Evidence-Based Approach to Interacting with Open
Student Models, International Journal of Artificial Intelligence
in Education, 17(3), 273-303.
|