Educational Data Minding (EDM) has been defined as “an
emerging discipline, concerned with developing methods for
exploring the unique types of data that come from educational
settings, and using those methods to better understand students,
and the settings which they learn in.” (International
Society of EDM).
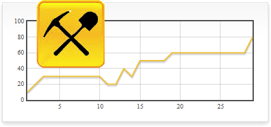 |
An extensive
overview of EDM applications, developments and definitions
has been given by Baker and Yacef (2009). EDM has its
origins in multiple research areas such as statistics,
data mining, machine learning, visualization and computational
modelling aiming to automatically discover patterns
and models from huge and extensively growing datasets.
While in the beginnings of EDM, most data was retrieved
from experimental learning sets not lasting longer than
a couple of weeks, nowadays such data is often tracked
over the duration of a whole course lasting up to one
study year. Collected data is further analysed to gain
valuable insights on learning processes and aspects.
With these enormous loads of data new
challenges arise
|
especially in visualizing and modelling the information in a
manner still readable and interpretable for human stakeholders.
While EDM aims to discover patterns and models on scaled data,
Learning Analytics (LA) further includes the needs of different
educational stakeholders and the strength of their judgement
in addition to computational measurements. The focus areas of
both communities (EDM and LA) seem to be slightly different,
although they follow similar goals that encompass the improvement
of educational technology and the evaluation of pedagogically
sound instructional designs (Baker et al., 2012).
Learning Analytics especially emphasises on supporting pedagogical
approaches by providing assistance to teachers in practically
relevant questions (e.g., the quality of learning material
or the engagement of students in specific exercises). Data
gained by LA tools can be used to evaluate pedagogically sound
instructional designs within classroom settings. In most cases
this focuses mainly on monitoring learner actions and their
interactions with learning tools and learning peers (Lockyer
& Dawson, 2011). Many attempts go towards visualizing
these learning traces in order to make significant relationships
explicit or even to allow stakeholders to discover such relations
independently. Research using dashboards in LAs has been described
by Duval (2011). As known in other domains like for instance
sports, the visualization of collected interaction training
data and the comparison with of this data with data of like-minded
peers, may lead to insights about unwelcome habits and better
practices but also to higher motivation due to the playful
introduction of competitiveness. Dychkoff et al. (2012) describe
a learning analytics toolkit that takes a step forward by
allowing the course instructors to define their own learning
indicators via combining traced student actions. This toolkit
is designed to support the instructors in optimizing their
teaching strategies. An example of inferences drawn from the
learning indicators about the quality of teaching material
is the relation between time spent on specific learning objects
and learning outcome. Also hardly touched learning objects
can be assumed of poor quality with respect to the learning
audience.
The future of TEL is often seen in flexible, teacher or
even learner composed mash-up learning frameworks, independent
of a specific environment (Chatti et al., 2010). To keep up
with this development new learning analytics toolkits need
to be just as flexible and adaptable as learning tools.
|
|
References
Baker, R. S. J. D., Duval, E., Stamper, J., Wiley, D., & Buckingham-Shum,
S. (2012). Social Learning Analytics: Five Approaches. In Proceedings
of the 2nd International Conference on Learning Analytics and Knowledge
(20-20). New York: ACM.
Baker, R. S. J. D., & Yacef, K. (2009). The State of Educational
Data Mining in 2009: A Review and Future Visions, Journal of Educational
Data Mining, 1(1), 3-17.
Chatti, M. A., Agustiawan, M. R., Jarke, M., & Specht, M. (2010).
Toward a Personal Learning Environment Framework. International
Journal of Virtual and Personal Learning Environments, 1(4), 66-85.
Duval, E. (2011). Attention please!: learning analytics for visualization
and recommendation. In Proceedings of the 1st International Conference
on Learning Analytics and Knowledge (9-17). New York: ACM.
Dyckhoff, A. L., Zielke, D., Bültmann, M., Chatti, M. A., &
Schroeder, U. (2012). Design and Implementation of a Learning Analytics
Toolkit for Teachers. Journal of Educational Technology & Society,
15(3), 58-67.
Lockyer, L. & Dawson, S. (2011). Learning designs and learning
analytics. In In Proceedings of the 1st International Conference
on Learning Analytics and Knowledge (153-156). New York: ACM.
|