One of the main aims of LEA’s BOX is to provide a competence-centred
and non-invasive methodology for the assessment of the learning
progress of individual learners as well as groups of learners.
The notion of learning progress implies the change of a learner´s
current state of knowledge, abilities, skills and competences
over time. A valid assessment of such changes over time, or
in other words, a valid and non-invasive assessment of learning
by means of Learning Analytics, requires a precise and well-described
representation of the learning domain. LEA´s BOX applies
two psycho-pedagogically sound frameworks to describe the
learning domain in a formalized and precise way: The Formal
Concept Analysis and the (Competence-based) Knowledge Space
Theory.
Another framework with a similar mathematical background,
definitions, and objectives is the Competence-based Knowledge
Space Theory which provides a theoretical framework for knowledge
and competence modeling (Albert & Lukas, 1999; Falmagne
& Doignon, 2011; Falmagne, Albert, Doble, Eppstein, &
Hu, 2013). It is a powerful approach for structuring and representing
domain and learner knowledge. In its original formalisation,
a knowledge domain is characterized by a set of problems or
test items. The knowledge state of an individual is identified
with the subset of problems this person is able to solve.
Due to mutual dependencies between the problems, not all potential
knowledge states will occur. These dependencies are captured
by the so-called prerequisite relation or its generalisation,
the prerequisite function. The collection of all possible
states is called a knowledge structure.
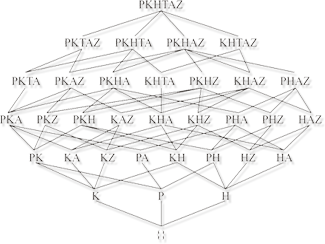 |
Competence-based
extensions of the original framework (Albert & Lukas,
1999; Heller, Ünlü, & Albert, 2013; Heller,
Steiner, Hockemeyer, & Albert, 2006) consider the
latent cognitive constructs underlying observable behaviour
and assume a competence structure on a set of abstract
skills underlying the problems and learning objects
of the domain. By associating skills to the problems
and learning objects of a domain, knowledge and learning
structures on the problems and, respectively, learning
objects are induced. The skills, which are not directly
observable, can be uncovered on the basis of a person’s
observable performance. Skills are thereby commonly
defined adopting learning and teaching goals as they
can be identified from the curriculum (Korossy, 1997)
and by combining action/procedural and conceptual/declarative
components (Marte, Steiner, Heller, & Albert, 2008).
These skills can be related to existing educational
taxonomies (e.g. Anderson & Krathwohl, 2001); the
skill modelling approach of CbKST is therefore in line
with approaches aiming at the standardised and comparable
representation of competence as an outcome of educational
programs or school types and at providing a supporting
frame for competence-oriented and learner-centred instruction
(e.g. BMUKK, 2012; European Communities, 2007, 2008;
European Commission, 2012).
|
The structures
CbKST formulates on skills (or problems) in terms of prerequisite
relations or functions can be graphically depicted by
Hasse diagrams (e.g. Pemmaraju & Skiena, 1990) and,
respectively, And/Or graphs, which are directed graphs
with the nodes representing the problems of a domain and
the arcs representing prerequisite relationships among
those problems. These structures are traditionally been
used at the backend of learning technologies, as a basis
for adaptation mechanisms. In the iClass project an approach
of opening the structures on domain skills and their association
with learning objects and assessment problems to end users
has been taken. A range of visual tools has been developed
to empower learners and teachers in planning and performing
their learning and teaching, and to help them in reflecting
on the learning and teaching process (Nussbaumer, Steiner,
& Albert, 2008; Steiner, Nussbaumer, & Albert,
2009). In particular, one of these tools – in line
with ideas of open learner models - visualises assessment
results on skills and reports them back to learners (and
teachers) to enable reflection on acquired skills and
identification of existing competence gaps. |
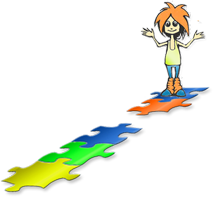
|
CbKST provides the basis for adaptive assessment procedures
of a learner’s current competence and knowledge state
as well as for the realisation of intelligent educational
adaptation and has been successfully applied as a cognitive
basis for realising in terms of personalising learning experiences
in different learning systems (Albert, Hockemeyer, & Wesiak,
2002; Conlan, O’Keeffe, Hampson, & Heller, 2006;
Falmagne, Cosyn, Doignon, & Thiéry, 2006). The
so-called microadaptivity approach (Augustin, Hockemeyer,
Kickmeier-Rust, & Albert, 2011; Kickmeier-Rust & Albert,
2010) has been developed and applied in the context of game-based
learning (Kickmeier-Rust, Mattheiss, Steiner, & Albert,
2011) and integrates CbKST with theory of human problem solving
(Newell & Simon, 1972) in order to model learners’
behaviour and skills in problems solving during learning and
assessment situations. The approach enables non-invasive assessment
of learners’ available and lacking skills by monitoring
and interpreting their (inter)actions in the learning environment
during problem solving and the gathered assumptions on a learner’s
skills serve the provision of adaptive hints, prompts or feedback
tailored to the learner’s available and lacking skills
(e.g. Kickmeier-Rust, Steiner, & Albert, 2011). Microadaptivity
can therefore be understood as an approach to formative assessment
and tailored educational interventions.
Formal Concept Analysis (FCA), established by Wille (1982),
aims to describe concepts and concept hierarchies in mathematical
terms. The starting point of the FCA is the specification
of a “formal context” (also called learning domain).
The formal context K is defined as a triple (G, M, I) with
G as a set of objects which belong to the learning domain,
M as a set of attributes which describe the learning domain,
and finally, I as a binary relation between G and M. The relation
I connects objects and attributes, i.e. (g, m) ∈ I means the
object g has the attribute m. The formal context K can be
best read when depicted as a cross table, with the objects
in the rows, the attributes in the columns and relations between
them by assigning “X” in the according cells.
A formal concept
is a pair (A, B) with A as a subset of objects and B
as a subset of attributes. A is called the extension
of the formal concept; it is the set of objects which
belong to the formal concept. B is called the intension,
it is the set of attributes which apply to all objects
of the extension. The ordered set of all formal concepts
is called the concept lattice B(K) (see Wille, 2005)
Every node of the Concept Lattice represents a single
formal concept. The extension of a particular formal
concept can be read off from the lattice by gathering
all objects which can be reached by descending paths
from that node. The intension is represented by all
attributes which can be reached by an ascending path
from that node. For example, the node with the label
“Leech” represents a formal concept with
{Leech, Goldfish) as extension and {m1, m2} as intension.
|
Learning Domain Biotope (Formal
Context based on Ganter and Wille, 1996)
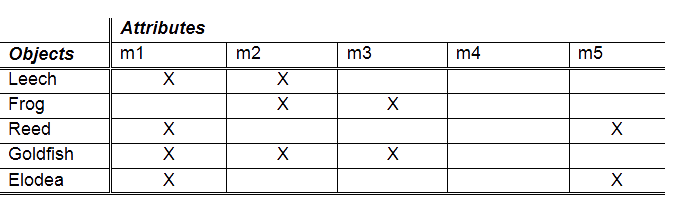
Notes
regarding attributes: m1…lives solely in the water,
m2…is able to change location,
m3… has limbs, m4…breastfeeds descendants,
m5…applies photosynthesis |
|
|
References
lbert, D., Hockemeyer, C., & Wesiak, G. (2002). Current trends
in eLearning based on knowledge space theory and cognitive psychology.
Psychologische Beiträge, 44, 478-494.
Albert D. & Lukas J. (1999). Knowledge spaces: Theories, empirical
research, applications. Mahwah: Lawrence Erlbaum Associates.
Albert, D., Nussbaumer, A. & Steiner, C.M. (2010). Towards Generic
Visualisation Tools and Techniques for Adaptive E-Learning, In S.L.
Wong et al. (Eds). International Conference on Computers in Education
(pp. 61-65), Putrajaya, Malaysia, Asia-Pacific Society for Computers
in Education.
Anderson, L.W. & Krathwohl, D.R (Eds.) (2001). A taxonomy for
learning, teaching, and assessing: A revision of Bloom's taxonomy
of educational objectives. New York: Longman.
Augustin, T., Hockemeyer, C., Kickmeier-Rust, M., & Albert,
D. (2011). Individualized skill assessment in digital learning games:
Basic definitions and mathematical formalism. IEEE Transactions
on Learning Technologies, 4, 138-148.
BMUKK (2012). Kompetenzorientiertes Unterrichten an berufsbildenden
Schulen. Grundlagenpapier [Competence-oriented instruction at vocational
schools. White paper]. Wien: Bundesministerium für Unterricht,
Kunst und Kultur.
Conlan, O., O'Keeffe, I., Hampson, C., & Heller, J. (2006).
Using knowledge space theory to support learner modeling and personalization.
In T. Reeves & S. Yamashita (Eds.), Proceedings of World Conference
on E-Learning in Corporate, Government, Healthcare, and Higher Education
(pp. 1912-1919). Chesapeake: AACE.
European Communities (2007). Key competencies for lifelong learning:
European Reference Framework. Luxembourg: Office for Official Publications
of the European Communities.
European Communities (2008). Explaining the European Qualifications
Framework for Lifelong Learning. Luxembourg: Office for Official
Publications of the European Communities.
European Commission (2012). Assessment of key competencies in initial
education and training: Policy guidance. Commission staff working
document. SWD(2012) 371. Strasbourg.
Falmagne, J.-C., Albert, D., Doble, C., Eppstein, D. & Hu, X.
(Eds.) (2013). Knowledge spaces: Applications in education. New
York: Springer.
Falmagne, J.-C., Cosyn, E., Doignon, J.P., & Thiéry,
N. (2006). The assessment of knowledge, in theory and practice.
In R. Missaou & J. Schmidt (Eds.), Formal Concept Analysis.
4th International Conference, ICFCA. Lecture Notes in Artificial
Intelligence vol. 3874 (pp. 61-79). Berlin: Springer.
Falmagne, J.- C. & Doignon, J. P. (2011). Learning Spaces: Interdisciplinary
Applied Mathematics. New York: Springer Verlag.
Fruhmann, K., Nussbaumer, A., & Albert, D. (2010). A Psycho-Pedagogical
Framework for Self-Regulated Learning in a Responsive Open Learning
Environment. Proceedings of the International Conference eLearning
Baltics Science.
Ganter, B., & Wille, R. (1996). Formale Begriffsanalyse - Mathematische
Grundlagen. Heidelberg: Springer Verlag.
Heller, J., Ünlü, A., & Albert, D. (2013). Skills,
competencies, and knowledge structures. In J.-C. Falmagne, D. Albert,
C. Doble, D. Eppstein, & X. Hu (Eds.), Knowledge spaces: Applications
in education. Springer.
Heller, J., Steiner, C., Hockemeyer, C., & Albert, D. (2006).
Competence-based knowledge structures for personalised learning.
International Journal on E-Learning, 5, 75-88.
Kickmeier-Rust, M.D., & Albert, D. (in press). Using Hasse Diagrams
for Competence-Oriented Learning Analytics. To appear in Springer
LNCS.Kickmeier-Rust, M.D., & Albert, D. (2010). Micro adaptivity:
Protecting immersion in didactically adaptive digital educational
games. Journal of Computer Assisted Learning, 26, 95-105.
Kickmeier-Rust, M.D., Steiner, C.,M. & Albert, D. (2011). Apt
to adapt: Micro- and macro-level adaptation in educational games.
In T. Daradoumis, S. Caballé, A. Juan & F. Xhafa (Eds.),
Technology-Enhanced Systems and Tools for Collaborative Learning
Scaffolding. Studies in Computational Intelligence vol. 350 (pp.
221-238). Berlin: Springer.
Kickmeier-Rust, M.D., Mattheiss, E., Steiner, C.M., & Albert,
D. (2011). A psycho-pedagogical framework for multi-adaptive educational
games. International Journal of Game-Based Learning, 1. 45-58.
Korossy, K. (1997). Extending the theory of knowledge spaces: a
competence-performance approach. Zeitschrift für Psychologie,
205, 53-82.
Kopeinik, S., Nussbaumer, A., Bedek, M., Albert, D. (2012). Using
CbKST for Learning Path Recommendation in Game-based Learning. In
Proceedings of the 20th International Conference on Computers in
Education (pp. 86 – 91), ICCE 2012.
Marte, B., Steiner, C.M., Heller, J., & Albert, D. (2008). Activity-
and taxonomy-based knowledge representation framework. International
Journal of Knowledge and Learning, 4, 189-202.
Newell A. & Simon H. (1972) Human problem solving. Englewood
Cliffs, NJ: Prentice-Hall.
Nussbaumer, A., Steiner, C., & Albert, D. (2008). Visualisation
tools for supporting self-regulated learning through exploiting
competence structures. In K. Tochtermann, H. Maurer, F. Kappe, &
W. Haas (Eds.), Proceedings of I-KNOW’08 and I-MEDIA’08.
International Conferences on Knowledge Management and New Media
Technology (pp. 288-295). Graz: J.UCS.
Papanikolaou, K. & Grigoriadou, M. (2003). Adaptive web-based
learning: accommodating individual differences through system’s
adaptation. British Journal of Educational Technology, 34(4), 511
-527.
R usch, A., & Wille, R. (1996). Knowledge spaces and formal
concept analysis. In H. H. Bock & W. Polasek (Eds.), Data analysis
and information systems: Statistical and conceptual approaches (pp.
427-436). Berlin: Springer.
Ünlü, A., Schrepp, M., Heller, J., Hockemeyer, C., Wesiak,
G., & Albert, D. (2013). Recent developments in performance-based
knowledge space theory. In J.-C. Falmagne, D. Albert, C. Doble,
D. Eppstein, & X. Hu (Eds.), Knowledge spaces: Applications
in education. New York: Springer.
Wille, R. (1982). Restructuring lattice theory: An approach based
on hierarchies of concepts. In I. Rival (Ed.), Ordered sets (pp.
445–470). Dordrecht: Reidel.
Wille, R. (2005). Formal Concept Analysis as Mathematical Theory
of Concepts and Concept Hierarchies, In B. Ganter , G. Stumme and
R. Wille (Eds.), Formal Concept Analysis (pp. 1-34), Berlin: Springer.
|